Faulty generalisation is a common fallacy – an error in an argument – in both academic and non-academic discourse. But what is faulty generalisation? And how do you avoid it in your work? Check out our guide to find out.
What Is Faulty Generalisation?
Faulty generalisation – sometimes known as hasty generalisation or defective induction – involves drawing a conclusion for an entire population based on a limited sample. In other words, we make a faulty generalisation when we jump to an unjustified conclusion. For instance:
My friend Bill is bald, so I assume nobody called Bill has hair.
In this case, our sample is Bald Bill. The population we’re generalising about is everyone called Bill. But this is obviously an unjustified leap!
There are many people called Bill, so assuming we can say anything about all of them based on a single hairless person is a hasty generalisation.
Faulty Generalisation: Is the Sample Big Enough?
Not all faulty generalisations are as obvious as the one above. For instance:
Our study found that 80% of felines prefer Meow brand cat food.
This sounds impressive because 80% is a large percentage. But unless we know how many cats were tested, we cannot generalise to the wider feline population. If the study included 10,000 cats, we might have a decent sample size to draw conclusions. But if they only tested five cats, we couldn’t say much about all cats based on what 80% (i.e. four cats) did.
Find this useful?
Subscribe to our newsletter and get writing tips from our editors straight to your inbox.
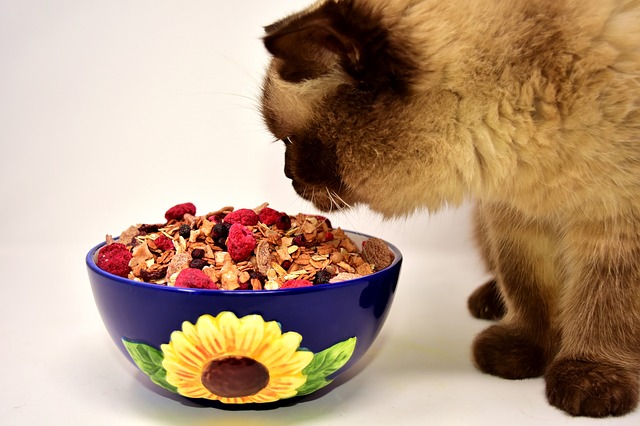
(Photo: Alexas_Fotos)
The key is that we should not jump to conclusions about large populations based on a limited sample size. And if we do not know the size of the sample in a study, we can’t know how reliable its conclusions are.
Faulty Generalisation: Is the Sample Representative?
In other cases, we need to look at whether the sample is representative of the wider population to which we are generalising. For example, imagine we conducted another survey about house cats:
In a study of 50,000 domestic felines, we found that 91% regularly climb trees. This suggests that the instinct to climb trees is strong in all felines.
A rate of 91% in a sample of 50,000 is impressive, so this could sound convincing. But are we sure domestic cats are representative of other felines? A house cat has some things in common with a lion, but it is also different in many ways. Generalising from one to the other would ignore these differences. Lions, for example, rarely climb trees.
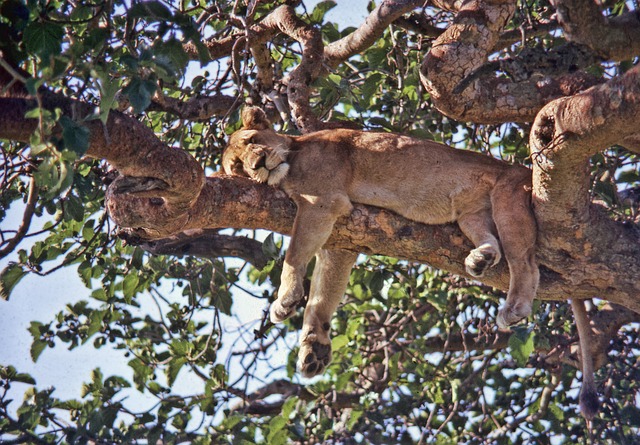
(Photo: Graham-H)
In this case, then, we need to be careful about generalising not because the sample is too small, but because we can’t be sure it reflects the wider population to which we are generalising.
How to Avoid Faulty Generalisations
None of the above means we cannot make generalisations in our arguments. Inductive reasoning – i.e. drawing probable conclusions based on evidence – is vital in many fields of study. But we need to draw conclusions based on reliable evidence. Thus, to avoid faulty generalisations, you should:
- Make sure generalisations are based on a large enough sample. You can use statistical tools to work out the minimum sample size required.
- Use a sample that is representative of the wider population. The sampling techniques you use will be important in this respect.
- If you are using someone else’s data, always check how they selected a sample before applying their results to a wider population.
In summary, make sure you don’t generalise based on a small or unrepresentative sample. Having your documents proofread can also help you to express your arguments as clearly as possible, thus preventing misunderstandings from arising when you make generalisations.